Background
The ICRA-DJI RoboMaster AI Challenge is a game of cooperation and competition between robots in a partially observable environment, quite similar to the Counter-Strike game. It had been hosted for three years by the RoboMaster Organizing Committee in conjunction with the IEEE International Conference on Robotics and Automation(ICRA). The ICRA-DJI RoboMaster AI Challenge 2019 was held from May 20th until May 22nd in Montreal, Canada. The competition had a total of 68 teams registered.
Our BayesianBot Team
I’m the captain of the team. Our team consists of two graduate students (I and Zhu Jiahui) and four undergrad students (Zhou Yang, Li Yike, Huang Jingyi and Nian Zhonglin) from the School of Information Science and Technology. Our team, led by Prof. Andre Rosendo, qualified in first place at the group stage and proceeded to the quarter finals, winning a “top 8 award” (called the 2nd Prize) of 2000 dollars. Among students in the team, Zhang Yizheng, Zhu Jiahui, Huang Jingyi and Nian Zhonglin are members of the LIMA Lab, where Bayesian Learning applied to Reinforcement Learning (very famous research topic after the success of AlphaGo) is the main research topic. Zhou Yang and Li Yike both attended the 2018 RISS summer research program held by the Robotics Institute of Carnegie Mellon University and got great benifits.
Technique
- Adaptive Monte Carlo Localization (AMCL) approach for the self-localizing problem.
- Combining a local planner (Time Elastic Band) and a global planner (A*) to move the robot to specific positions at the arena.
- For our computer vision strategy, we trained a Convolutional Neural Network with 240 images of the enemy robot, and used a lightweight implementation called YOLOv3, capable of running at high speed (more than 30 FPS) at a fast GPU-based microcontroller, called Nvidia TX2.
- From a decision-making perspective we created a Reinforcement Learning strategy by using a data-efficient Policy Search Algorithm which uses Bayesian Deep Learning to find optimal policies with very few trials. This strategy was also the reason why the team was named BayesianBot.
Photo Gallery

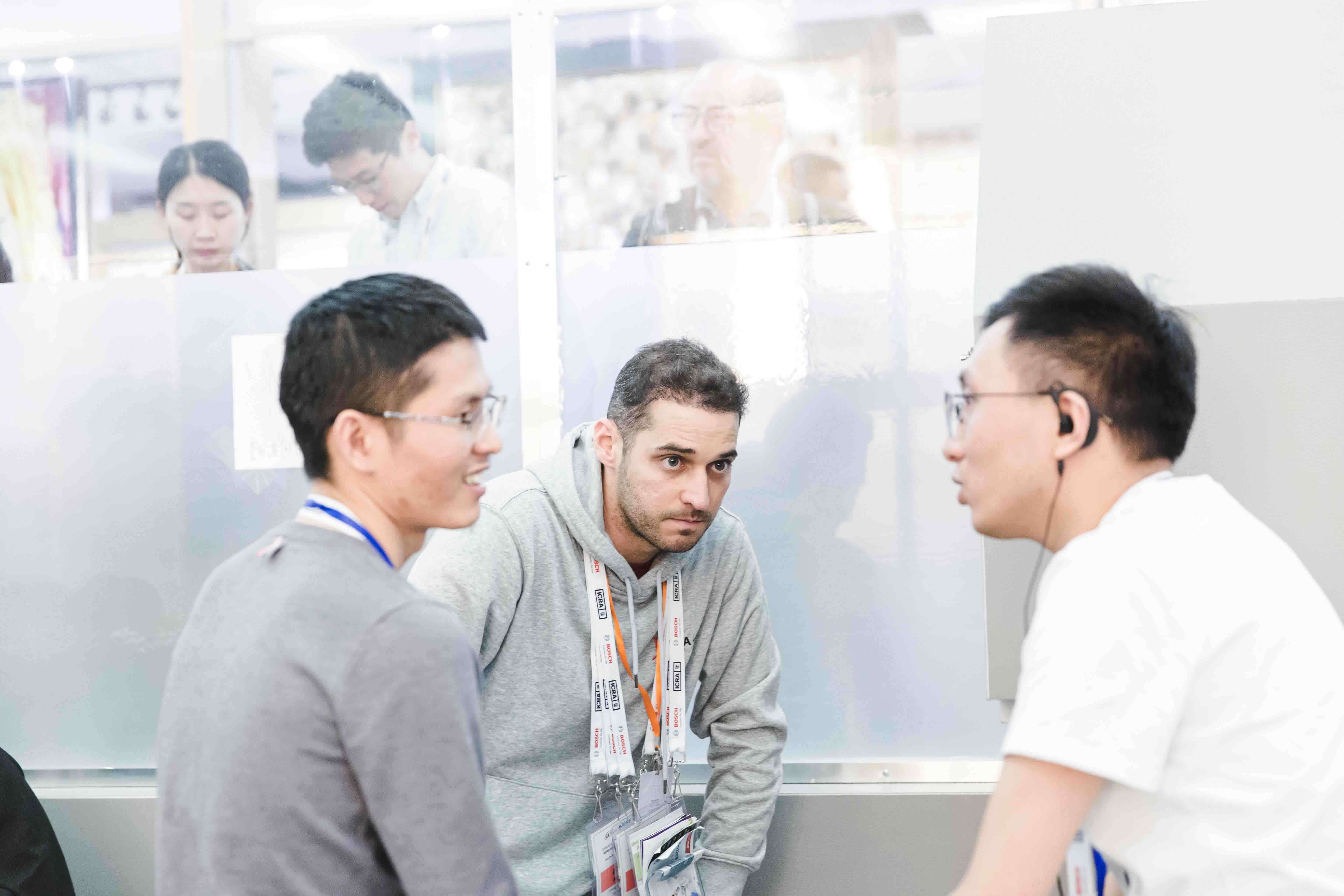


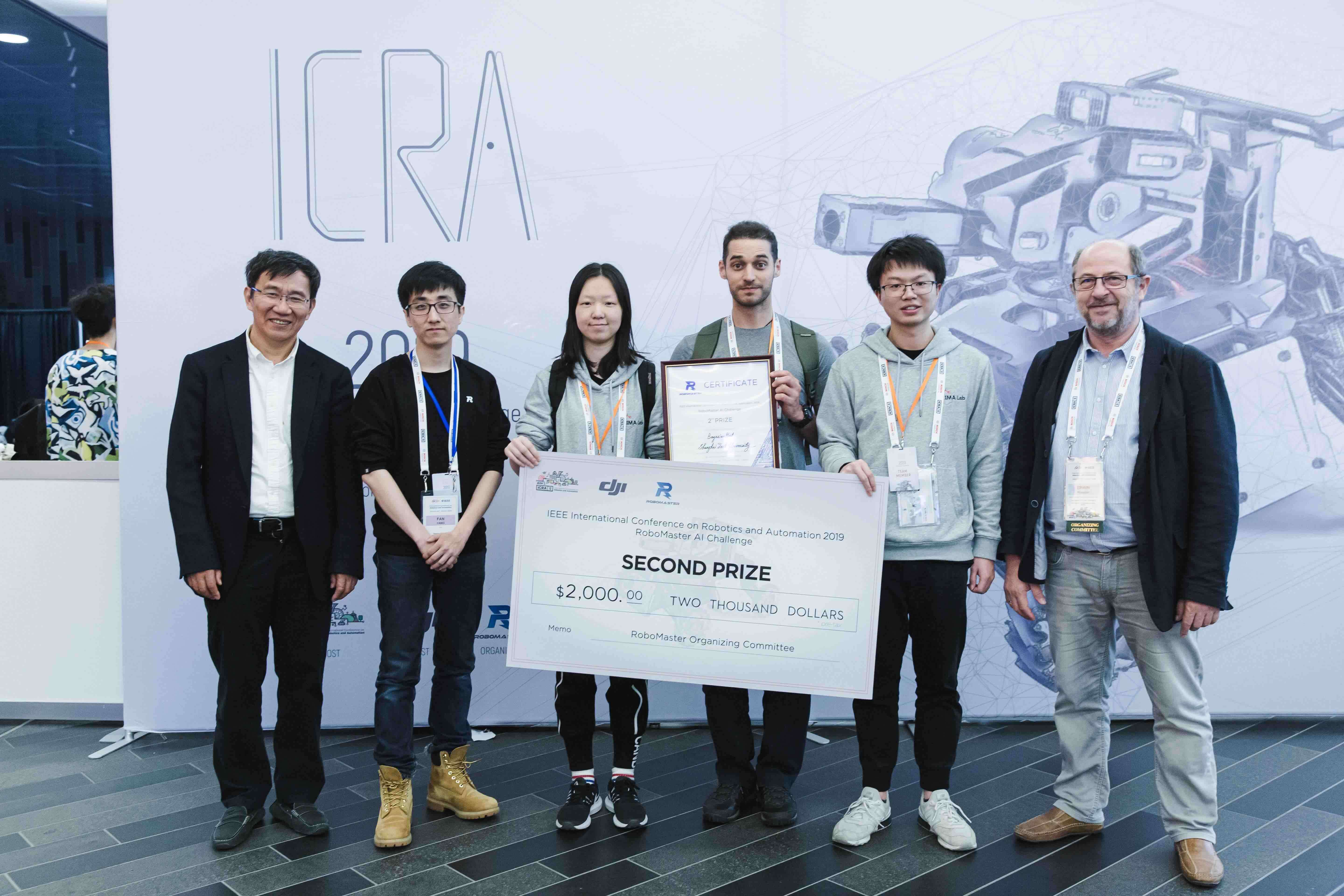
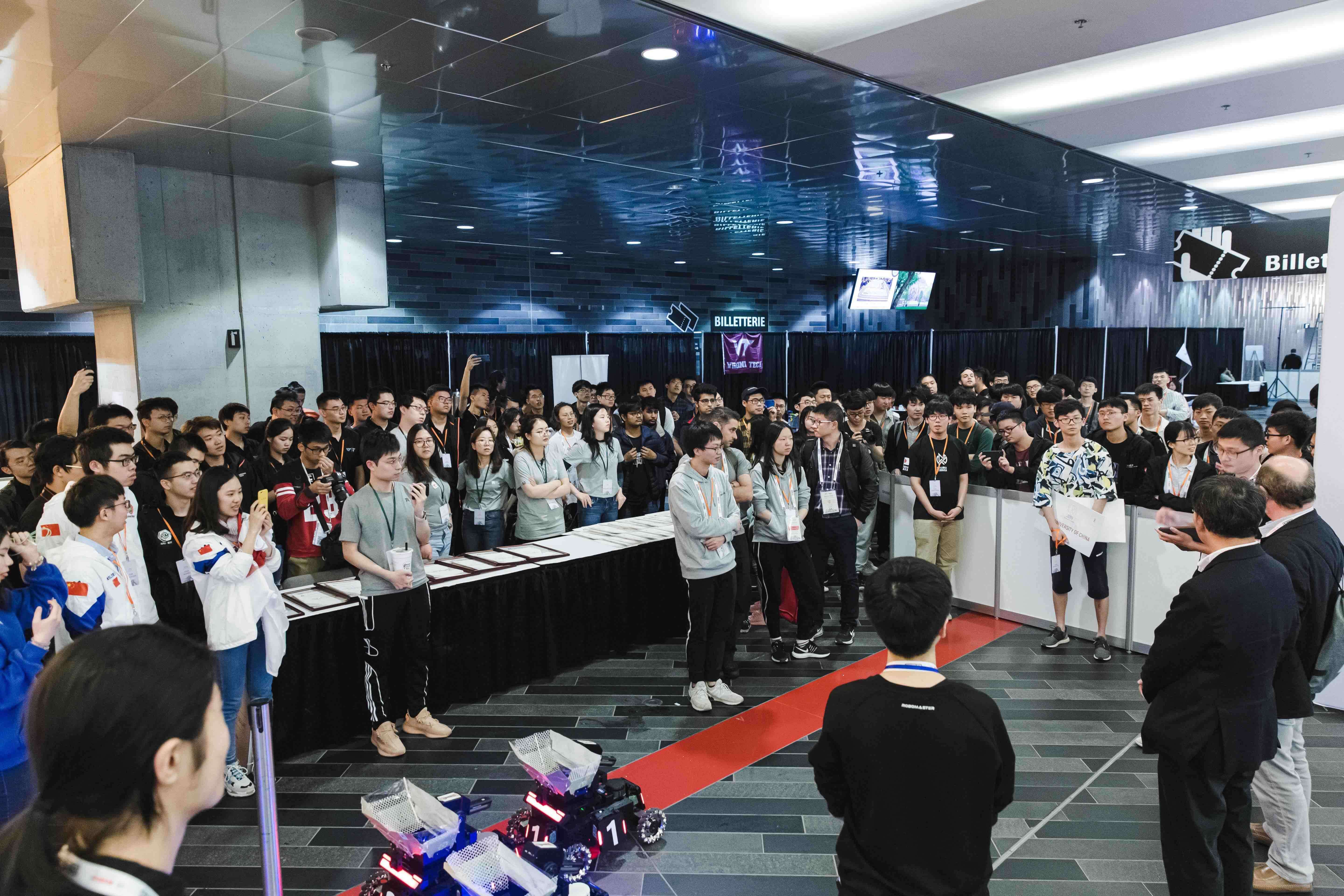
Competition Process
Before the competition started the teams had to write a report to qualify for the competition, and our team BayesianBot received an A in our report, qualifying in the 9th position. At the competition the team was placed in a group of 3 universities, qualifying in 1st place at the group and proceeding to the Quarter-finals. There, the team faced Zhejiang University and lost in a nail-biting match where Zhejiang won for 3 shots. Later, Zhejiang proceeded all the way to the final match, where it lost to Beijing Institute of Technology.
Technical Report Teaser
Technical Report Video
Paper
Tactical Reward Shaping: Bypassing Reinforcement Learning with Strategy-Based Goals